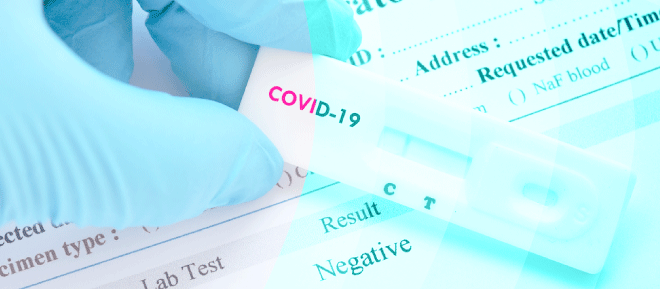
27 Oct The Challenges of Understanding Covid-Related Risk: Part B
In Part A of this article, we introduced the COVINFORM Dashboard. We talked about how the Risk Assessment framework presented in the Dashboard is composed of four domains: Threat, Vulnerabilities, Consequences, and Resilience, and how each of these domains are composed of multiple ‘indicators’. In Part B, we explore the challenges faced by Trilateral’s DARSI team while selecting, collecting and processing data that accurately represents these indicators.
The Challenges of Data Collection
The first major challenge that the team faced was finding data that accurately represented the indicator of interest.
Using expert advice, the team collated a list of key indicators, such as the examples above, that could contribute towards building a holistic understanding of the risks posed by the pandemic. However, understanding which data would most accurately reflect the indicators in question was sometimes challenging. The team decided on which data to include by conducting in-depth literature reviews, assessing data availability and investigating data quality.
The second major challenge was dealing with missing data within datasets. In some cases, data that accurately represented indicators of interest were straightforward to collect from published databases. However, in some cases, data was missing. For example, while the UK had survey data on how educational progress was impacted by the pandemic, these data were not available for EU countries. This meant that the team had to look at which data were available in EU countries and decide on what best aligned with the UK data. Identifying data gaps and selecting data that aligned well, despite being slightly different in what they measured or how they were measured, posed significant logistical and time challenges to the team.
Another major challenge was deciding how to aggregate data. The original data was available at different geographic scales (i.e., national and regional) and temporal scales (i.e., daily and annual), and needed to be aggregated into national-level, yearly data. Choosing the best method for conducting this aggregation was not straightforward, because the team wanted to ensure that they were not introducing inaccuracies or bias.A fourth major challenge was whether to collect quantitative or qualitative data. Where possible, the team wanted to collect quantitative data, because it is less likely to be subjective (i.e., a measurement based on someone’s perception rather than the direct measurement itself) and therefore is likely to be a more accurate reflection of reality. However, in some cases it was only possible to collect qualitative data.The Challenges of Data Processing
Following collection, the data had to be processed such that it was directly comparable across countries and time and could be meaningfully combined into a single score.
Most of the data was collected from Eurostat, which standardises data so that it can be compared between countries and across years. However, following Brexit, Eurostat no longer requested UK data, and some important indicators were therefore missing from the Eurostat database. As a consequence, the team had to collect data from multiple sources and figure out how to standardise the data so that it was comparable to Eurostat’s.
A particular difficulty was dealing with different measurements used in different datasets. For instance, the way population age is recorded is different between the UK and Eurostat: both aggregate the data into different age groups, but the group boundaries are different.
Data also had to be normalised so that it was directly comparable across countries. However, choosing the best normalisation strategy was not trivial; different normalisation techniques, while all valid, have assumptions that influence the results of downstream analysis.
Conclusions
As with any project that combines a large amount of different data types from a wide range of sources, difficult decisions had to be made at every level of data collection and processing to ensure that the information shown in the Dashboard and associated COVINFORM Risk Score is (1) an accurate representation of reality and (2) easily interpretable.
For the Dashboard to be as trustworthy, transparent and open as possible, the DARSI team have recorded the reasoning behind the data decisions they have made at every stage of the Dashboard’s development, and the source of all data used to build the COVINFORM Risk Score will be linked and referenced. Moreover, iterative workshops with End Users have helped the team develop a User Interface that is intuitive and straightforward, ensuring that the Dashboard can be used and interpreted with ease.
The COVINFORM Dashboard will be an invaluable research tool for academics, researchers and policymakers. It enables users to directly compare how countries were impacted by the COVID-19 pandemic across a wide range of metrics, with a focus on understanding how the pandemic influenced those who are most vulnerable in society. It may therefore help us identify the unique attributes of risk in the event of a future pandemic, and guide research into how to minimize the impact of societal inequality on pandemic-related health outcomes.